How Fairmarkit’s Supplier Recommendation Engine Optimally (and Instantaneously) Matches Requests and Suppliers
Fairmarkit is highly focused on supporting process improvements and efficiencies in procurement. From our autonomous sourcing suite that provides autonomous sourcing capabilities for tail spend to integrated decision and process support tools, it’s at the heart of what we do. But our tools are only as powerful as the models and algorithms behind them, which is why we invest tirelessly in technical improvements big and small. As we continue to develop our AI and ML suite, we will pull back the curtain to show what’s going on behind the scenes.
What is our supplier matching engine?
For each sourcing event, our supplier matching engine identifies the most relevant suppliers based on the goods and/or services being requested. By extension, it also identifies the most relevant opportunities for each supplier in our network. Taken in combination, the outcome is an optimal matching between requests and suppliers.
How is it helping our customers?
Our supplier matching engine is a building block that powers multiple pieces of functionality in the Fairmarkit platform. In the most direct application, recommended suppliers are displayed to buyers as they are building an RFQ or RFP. When used in this way, buyers frequently discover new suppliers that they would not otherwise have invited to participate in the sourcing event. In fact, based on the results of a large-scale A/B test, our customers received an average of 60% more quotes per event when presented with recommended suppliers versus when they assembled their supplier list manually. These additional quotes translate into cost savings and easier compliance. Our customers save an extra 6% when they use supplier recommendations versus when they don’t, and award more frequently to suppliers that contribute to strategic initiatives, such as ESG (Environmental, Social, and Governance) or DEI (Diversity, Equity and Inclusion).
Beyond this direct application, our supplier recommendations are an important input into our autonomous sourcing capabilities. For instance, automated bundling is triggered when similar suppliers are able to quote on all component goods or services in a given request; we identify this set of suppliers using our supplier matching engine. The same is true for our automated sending capability.
Finally, we utilize our matching engine to prioritize opportunities for suppliers in our network. In this way, our suppliers, like our buyers, are able to focus their effort on their highest value activities. The result is improved supplier engagement, fewer irrelevant requests for quotes, and more new business opportunities for our buyers and suppliers alike.
What powers it?
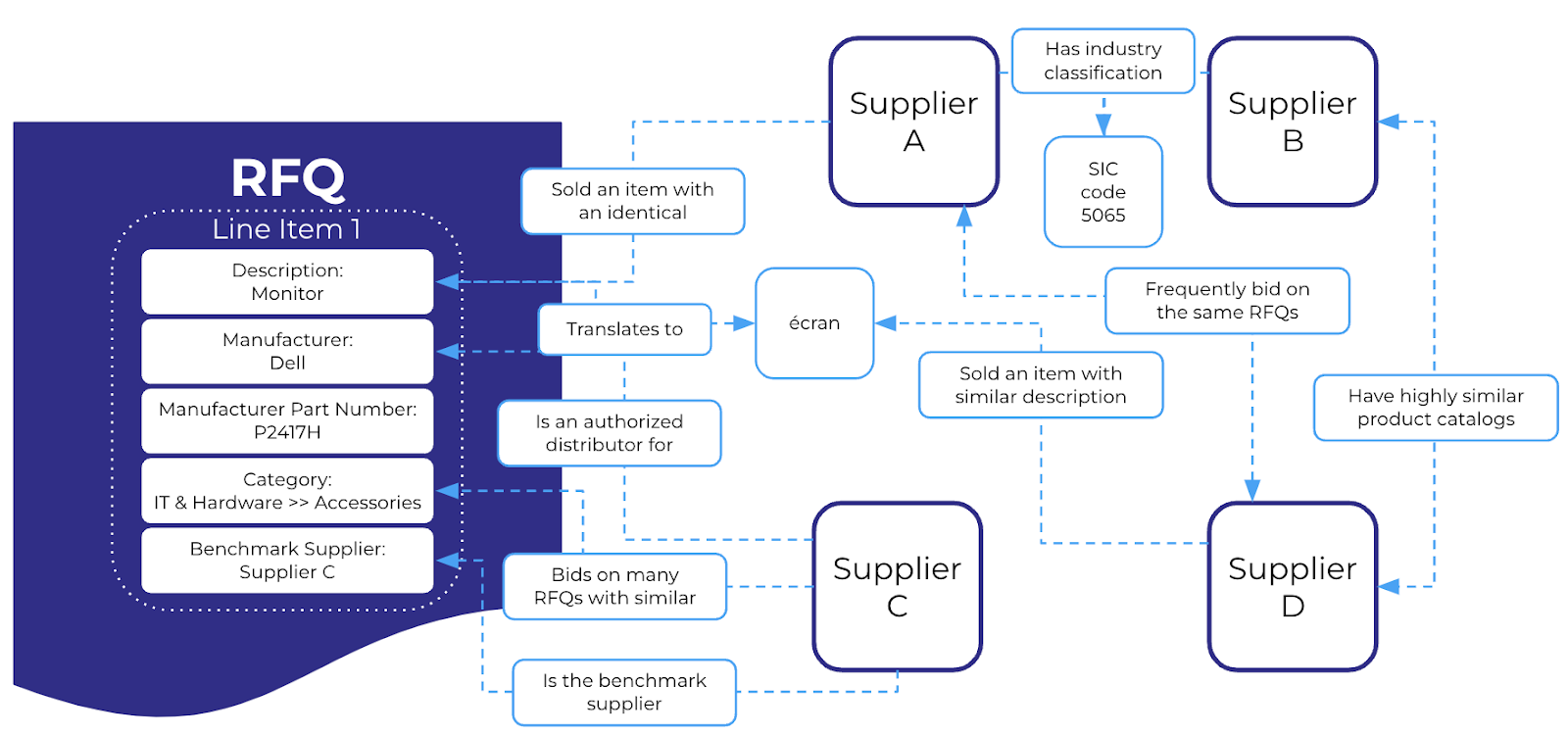
Our matching engine ingests all available information about each sourcing event as well as our suppliers’ capabilities using our advanced ML-based techniques. This model suite provides us with first degree matches – suppliers who are likely to quote on an opportunity based on their direct past activity, such as having sold an item in the same category previously or listing a large number of items from a given manufacturer in their catalog.
One major recent innovation has been to identify suppliers indirectly based on their position in our supplier graph, which represent second (or higher) degree matches. In this case, we may not have any historical data on supplier B having sold the item(s) being requested, but perhaps we know that supplier B and supplier A are highly similar based on industry and product classifications while suppliers D and A are highly similar because they frequently quote on the same RFQs within a given spend category. In this case, we are able to match suppliers B and D with the RFQ only indirectly, which would not have previously been possible. Additionally, within the set of matched suppliers, this approach allows us to rank similar suppliers more highly – all other things equal, we have found that clustering and boosting highly similar suppliers results in significantly improved match relevancy.
In addition to identifying the most relevant suppliers for each event, we quantify supplier relevancy by predicting the probability that a supplier will provide a quote on each event. Any suppliers with low such probabilities are eliminated from the match results. We utilize these predictions as an indication of match quality for our users. For recommended suppliers, we present a confidence score between 1 and 5, with 5 being the most likely to respond. This value is calculated based on how the supplier company, including all of the supplier's individual contacts, responded to similar events and their current activity level in Fairmarkit.
How can I use it? Are there any best practices?
Our matching engine powers supplier recommendations, which are available on all RFQ or RFP events on the Fairmarkit platform. To use them, simply click the “Refresh Suppliers” button in the suppliers invitation area on the RFQ page. It may take several seconds to receive results – we have an extensive supplier network comprising hundreds of thousands of suppliers, tens of millions of unique goods and services, and hundreds of billions of dollars of spend analyzed.
In order to maximize the performance of supplier recommendations, our recommended approach is to adhere to best practices as if you were using a search engine to search in any other capacity. Ensuring that the request includes all of the relevant context but more detailed requests will work best. If you are looking for a specific manufacturer, specify that in the corresponding field. For generic items, describe the item in the same way that your suppliers would. After all, once a supplier matches your sourcing event, that supplier needs to understand what exact goods and services are being requested!